来自 | 知乎 作者 | Superbrother
链接 | https://zhuanlan.zhihu.com/p/81350196
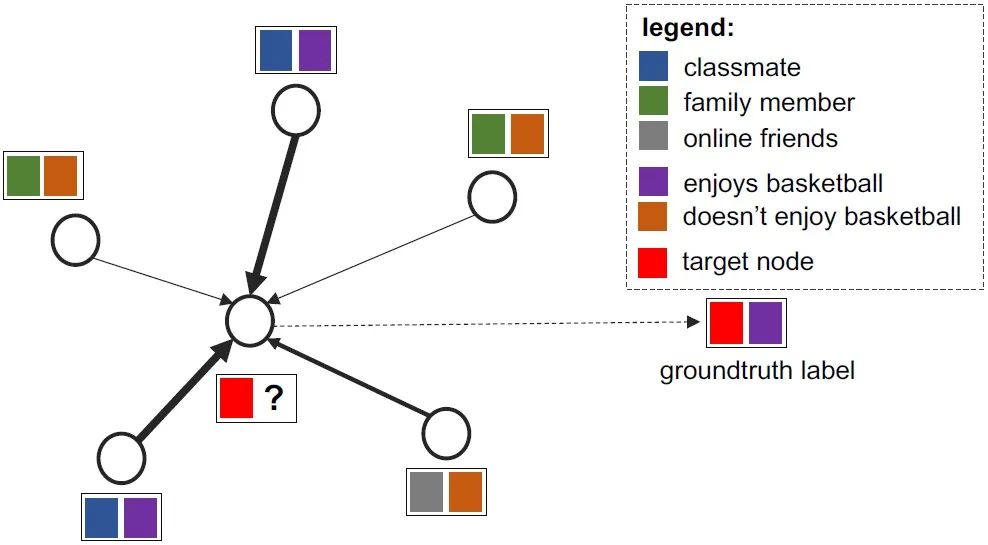
GRAPH ATTENTION NETWORKS的诞生
随着GCN的大红大紫,graph领域的deep learning研究可谓变得风生水起,人工智能又出现了新的网红。GCN在一系列任务取得了突破性进展的同时,一系列的缺点也逐渐被放大。
深度学习三巨头”之一的Yoshua Bengio组提出了Graph Attention Networks(下述简称为GAT)去解决GCN存在的问题并且在不少的任务上都取得了state of art的效果,是graph neural network领域值得关注的工作。
1 聊点基础
登堂入室之前,先介绍三点基础问题。
1.1 Graph数据结构的两种“特征”
当我们说起graph或者network的数据结构,通常是包含着顶点和边的关系。研究目标聚焦在顶点之上,边诉说着顶点之间的关系。
对于任意一个顶点 ,它在图上邻居
,构成第一种特征,即图的结构关系。

图1 graph示意图
当然,除了图的结构之外,每个顶点还有自己的特征 (通常是一个高维向量)。它可以使社交网络中每个用户的个体属性;可以是生物网络中,每个蛋白质的性质;还可以使交通路网中,每个交叉口的车流量。Graph上的deep learning方法无外乎就是希望学习上面的两种特征。
1.2 GCN的局限性
GCN是处理transductive任务的一把利器(transductive任务是指:训练阶段与测试阶段都基于同样的图结构),然而GCN有两大局限性是经常被诟病的:
(a)无法完成inductive任务,即处理动态图问题。inductive任务是指:训练阶段与测试阶段需要处理的graph不同。通常是训练阶段只是在子图(subgraph)上进行,测试阶段需要处理未知的顶点。(unseen node)
(b)处理有向图的瓶颈,不容易实现分配不同的学习权重给不同的neighbor。
1.3 Mask graph attention or global graph attention
还有一件事件需要提前说清楚:GAT本质上可以有两种运算方式的,这也是原文中作者提到的
-
Global graph attention
-
Mask graph attention


<pre style="max-width: 100%;box-sizing: border-box !important;overflow-wrap: break-word !important;"><section style="margin-right: 8px;margin-left: 8px;max-width: 100%;letter-spacing: 0.544px;white-space: normal;color: rgb(0, 0, 0);font-family: -apple-system-font, system-ui, "Helvetica Neue", "PingFang SC", "Hiragino Sans GB", "Microsoft YaHei UI", "Microsoft YaHei", Arial, sans-serif;widows: 1;line-height: 1.75em;box-sizing: border-box !important;overflow-wrap: break-word !important;"><strong style="max-width: 100%;box-sizing: border-box !important;overflow-wrap: break-word !important;"><span style="max-width: 100%;letter-spacing: 0.5px;font-size: 14px;box-sizing: border-box !important;overflow-wrap: break-word !important;"><strong style="max-width: 100%;font-size: 16px;letter-spacing: 0.544px;box-sizing: border-box !important;overflow-wrap: break-word !important;"><span style="max-width: 100%;letter-spacing: 0.5px;box-sizing: border-box !important;overflow-wrap: break-word !important;">—</span></strong>完<strong style="max-width: 100%;font-size: 16px;letter-spacing: 0.544px;box-sizing: border-box !important;overflow-wrap: break-word !important;"><span style="max-width: 100%;letter-spacing: 0.5px;font-size: 14px;box-sizing: border-box !important;overflow-wrap: break-word !important;"><strong style="max-width: 100%;font-size: 16px;letter-spacing: 0.544px;box-sizing: border-box !important;overflow-wrap: break-word !important;"><span style="max-width: 100%;letter-spacing: 0.5px;box-sizing: border-box !important;overflow-wrap: break-word !important;">—</span></strong></span></strong></span></strong></section><section style="max-width: 100%;letter-spacing: 0.544px;white-space: normal;font-family: -apple-system-font, system-ui, "Helvetica Neue", "PingFang SC", "Hiragino Sans GB", "Microsoft YaHei UI", "Microsoft YaHei", Arial, sans-serif;widows: 1;box-sizing: border-box !important;overflow-wrap: break-word !important;"><section powered-by="xiumi.us" style="max-width: 100%;box-sizing: border-box !important;overflow-wrap: break-word !important;"><section style="margin-top: 15px;margin-bottom: 25px;max-width: 100%;opacity: 0.8;box-sizing: border-box !important;overflow-wrap: break-word !important;"><section style="max-width: 100%;box-sizing: border-box !important;overflow-wrap: break-word !important;"><section style="max-width: 100%;letter-spacing: 0.544px;box-sizing: border-box !important;overflow-wrap: break-word !important;"><section powered-by="xiumi.us" style="max-width: 100%;box-sizing: border-box !important;overflow-wrap: break-word !important;"><section style="margin-top: 15px;margin-bottom: 25px;max-width: 100%;opacity: 0.8;box-sizing: border-box !important;overflow-wrap: break-word !important;"><section style="max-width: 100%;box-sizing: border-box !important;overflow-wrap: break-word !important;"><section style="margin-right: 8px;margin-bottom: 15px;margin-left: 8px;padding-right: 0em;padding-left: 0em;max-width: 100%;color: rgb(127, 127, 127);font-size: 12px;font-family: sans-serif;line-height: 25.5938px;letter-spacing: 3px;box-sizing: border-box !important;overflow-wrap: break-word !important;"><span style="max-width: 100%;color: rgb(0, 0, 0);box-sizing: border-box !important;overflow-wrap: break-word !important;"><strong style="max-width: 100%;box-sizing: border-box !important;overflow-wrap: break-word !important;"><span style="max-width: 100%;font-size: 16px;font-family: 微软雅黑;caret-color: red;box-sizing: border-box !important;overflow-wrap: break-word !important;">为您推荐</span></strong></span></section><section style="margin: 5px 8px;padding-right: 0em;padding-left: 0em;max-width: 100%;min-height: 1em;font-family: sans-serif;letter-spacing: 0px;opacity: 0.8;line-height: normal;box-sizing: border-box !important;overflow-wrap: break-word !important;"><span style="max-width: 100%;color: rgb(87, 107, 149);font-size: 14px;box-sizing: border-box !important;overflow-wrap: break-word !important;">人工智能领域最具影响力的十大女科学家</span><br style="max-width: 100%;box-sizing: border-box !important;overflow-wrap: break-word !important;" /></section><section style="margin: 5px 8px;padding-right: 0em;padding-left: 0em;max-width: 100%;min-height: 1em;font-family: sans-serif;letter-spacing: 0px;opacity: 0.8;line-height: normal;box-sizing: border-box !important;overflow-wrap: break-word !important;"><span style="max-width: 100%;color: rgb(87, 107, 149);font-size: 14px;box-sizing: border-box !important;overflow-wrap: break-word !important;">MIT最新深度学习入门课,安排起来!</span></section><section style="margin: 5px 8px;padding-right: 0em;padding-left: 0em;max-width: 100%;min-height: 1em;font-family: sans-serif;letter-spacing: 0px;opacity: 0.8;line-height: normal;box-sizing: border-box !important;overflow-wrap: break-word !important;"><span style="max-width: 100%;color: rgb(87, 107, 149);font-size: 14px;box-sizing: border-box !important;overflow-wrap: break-word !important;">有了这个神器,轻松用 Python 写个 App</span></section><section style="margin: 5px 8px;padding-right: 0em;padding-left: 0em;max-width: 100%;min-height: 1em;font-family: sans-serif;letter-spacing: 0px;opacity: 0.8;line-height: normal;box-sizing: border-box !important;overflow-wrap: break-word !important;"><span style="max-width: 100%;color: rgb(87, 107, 149);font-size: 14px;box-sizing: border-box !important;overflow-wrap: break-word !important;">「最全」实至名归,NumPy 官方早有中文教程</span><br style="max-width: 100%;box-sizing: border-box !important;overflow-wrap: break-word !important;" /></section><section style="margin: 5px 8px;padding-right: 0em;padding-left: 0em;max-width: 100%;min-height: 1em;font-family: sans-serif;letter-spacing: 0px;opacity: 0.8;line-height: normal;box-sizing: border-box !important;overflow-wrap: break-word !important;"><span style="max-width: 100%;color: rgb(87, 107, 149);font-size: 14px;box-sizing: border-box !important;overflow-wrap: break-word !important;">我为什么鼓励你读计算机领域的博士?</span></section><p style="margin-right: 8px;margin-bottom: 5px;margin-left: 8px;padding-right: 0em;padding-left: 0em;max-width: 100%;min-height: 1em;color: rgb(127, 127, 127);font-size: 12px;font-family: sans-serif;line-height: 1.75em;letter-spacing: 0px;box-sizing: border-box !important;overflow-wrap: break-word !important;"><br style="max-width: 100%;box-sizing: border-box !important;overflow-wrap: break-word !important;" /></p></section></section></section></section></section></section></section></section>
本篇文章来源于: 深度学习这件小事
本文为原创文章,版权归知行编程网所有,欢迎分享本文,转载请保留出处!
内容反馈